Submissions for the Critical Analysis category should critically evaluate or analyze a piece of literature, a theatrical performance, a work of visual art, a historical moment, a philosophical argument, a social movement, etc. Submissions should not exceed 20 pages. Amelia Jobe wrote the 1st place submission in the Critical Analysis Category for the 2025 President’s Writing Awards.
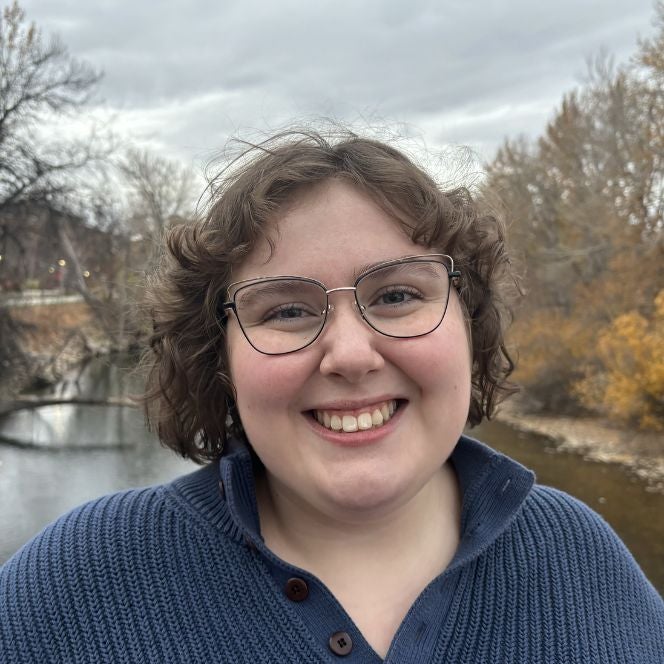
About Amelia
Amelia Jobe is a fourth-year student at Boise State University pursuing a BS in Sociology with minors in Gender Studies, Ethnic Studies, and Computer Science. They are deeply passionate about sociological research, with a particular interest in how technology intersects with other identities and institutions to shape systems of oppression. For the last two years, Amelia has been a researcher and mentor in the Intermountain Social Research Lab (IMSRL). There, she researches the impact of gender and neoliberal precarity on student experiences in higher education. This year, she also became a researcher in the Social Community Research Lab (SCRL). In addition to their research with IMSRL and SCRL, they have also conducted research on the gendered language of tech job postings, the impact of generative AI on collaboration in academic settings, and the impact of free breakfast on K-12 students. Outside of Sociology, she enjoys spending time with loved ones, snuggling with her cat Jahoda, cooking, and baking. She is honored to be selected for this year’s Presidential Writing Awards, and thanks you for reading her work.
Winning Manuscript – Marx Was not Prepared for Pornhub: Updating Alienation for a World with AI-Generated Porn
AI-generated pornography is an emerging technology that allows for the creation of new porn without the need for human actors. A particularly troubling type of AI-generated porn is the deep fake, which allows users to replace the face of an actor in a porn video with another person’s face with the click of a button (Wagner and Blewer, 2019). To understand the sociological impact of AI-generated porn, I turn to Marx’s concept of alienation. While this concept helps dissect the relationship between labor, exploitation, and the worker, it needs to be updated for a world with artificial intelligence, access to unfathomable levels of data, and professional, amateur, and AI-generated porn that continues to grow by the day. To refine this concept and apply alienation today, I have created the concept of AI-generated alienation.
AI-generated alienation is when one’s existence and humanity, captured through their digital footprint, is fed into an algorithm to generate and improve AI models. This process turns one’s digital footprint into a product of labor, whether that digital footprint was created to be labor or not. This digitized labor is fed into a deep-learning AI model, which further transforms this digital labor into a point in data. The result of this process is 1) a turning of one’s life into labor and then a digital product to be mined and purchased, and 2) this process obscures the sources that go into the final AI model project, which makes it impossible to determine the source of which the AI model emerges from. To this point, I argue that the generation, consumption, and lack of regulations surrounding AI-generated pornography leads to AI- generated alienation.
Before applying alienation to AI-generated porn, we must first revisit Marx’s theory of alienation. In the 1844 manuscripts, Marx describes his initial formulation of alienation, writing:
“The alienation of the worker in his product means not only that his labor becomes an object, an external existence, but that it exists outside him, independently, as something alien to him, and that it becomes a power of its own confronting him; it means that the life which he has conferred in the object confronts him as something hostile and alien.”
Marx writes that alienation creates an estrangement from the person and their labor. This estrangement turns the person’s labor into a product separable from them. The person’s labor becomes a commodity, separate, distinct, and obscured from its source of creation, with power over the original creator. While Marx’s concept of alienation is helpful when analyzing class- based power dynamics in a capitalist society, his idea of alienation was centered around the physical production of products. In the world of AI and the internet, one’s labor may result in a commodity that is not physically tangible. Digitization changes how one’s labor is shared and how it can be used and manipulated by others without the creator’s consent. In light of these circumstances, it is clear that Marx’s framework of alienation needs to be updated.
To understand the concept of AI-generated alienation, it is necessary to understand how AI video generation works. Deep learning models, the dominant AI framework today, work by identifying patterns in large amounts of data and then using these patterns to generate content that did not exist before. In the “training” portion of AI model generation, data is fed into the program, which allows the computer to “learn” patterns and meanings from the data, which can then generate new content (Wagner and Blewer, 2019). In the case of AI-generated porn, these deep learning systems are fed large amounts of pornography so that the programs can generate “original” porn.
Framing AI as generating “original” or “new” content is also misleading. The programs that generate content do not produce new, novel ideas, but rather produce an amalgamation of information. In other words, any AI video that is consumed is not new, but it is built upon a vast database of human labor; the only thing that is “new” about the technology is that it combines the labor of all the people who created the data, turns that labor into a new commodity, and makes it impossible to determine the source of the video.
Whereas “traditional” porn depicts the people in the porn, preventing the alienation of the actor, AI porn obscures the people in these videos, which separates the person from their labor. Thus, through the employment of AI, pornography is producing alienation by separating the labor of porn from the people in the videos.
Another troubling factor related to AI-generated alienation is that the end user cannot retrace or determine what pornographic videos the AI model was trained on and what conditions the actors in these videos participated in. Large free porn sites like Pornhub are notorious for hosting videos of rape, sexual assault, and child pornography, among other troubling categories. In 2017, Pornhub received over 4 million video uploads, which totaled 68 years of footage uploaded in just that year alone (Wagner and Blewer, 2019). The sheer volume of videos uploaded to these porn sites makes it nearly impossible to filter out videos that depict rape, sexual assault, child pornography, or revenge porn. Because AI porn models rely on being trained on high volumes of data, these harmful videos are being incorporated into AI-generated porn. To put it plainly: any AI-generated porn consumed is also an indirect consumption of child pornography, rape, and sexual assault, where the victim of these crimes is erased and turned into a data point to improve the AI algorithm.
Something that differentiates AI-generated alienation from Marx’s traditional formation of alienation is one’s consent in the use of their body in labor creation. As stated above, the “labor” or “commodity” being produced in pornographic videos may or may not be consensual, but nevertheless, the process of AI-driven alienation turns one’s presence in porn (or simply one’s digital footprint) into “labor.” Through the process of AI-driven alienation, one’s labor and digital footprint are violently separated from the creator and turned into an object of consumption without the creator’s consent.
What makes AI-generated porn particularly useful for understanding AI-generated alienation is the fact that the porn industry is built on a system of gender exploitation, with the field systematically reducing women to sexual objects, even if individual porn videos are made ethically (Öhman, 2020). Thus, the final product of AI porn is built on the exploitation of people in the porn industry, the exploitation of one’s digital footprint for AI-driven financial and social gains, and the alienation and obscuration of the original creators in the video from the final product and consumer.
Through the analysis of AI-generated porn, it is clear that Marx’s concept of alienation is a useful tool for understanding an emerging form of exploitation driven by data and AI. My concept of AI-generated alienation updates Marx’s theory of alienation. Thus, I argue that the generation, consumption, and lack of regulations surrounding AI-generated pornography leads to AI-generated alienation. By framing my concept around the topic of AI-generated porn, I highlight the ways that AI-generated alienation can be built on a system of gendered, sexual, and digital exploitation. The opaque process of AI video generation prevents the end-user from seeing the potentially coercive or nonconsensual situations in which these pornographic videos were produced, which alienates the actors (or in nonconsensual cases, victims) from their labor (either forced or consensual). This super-sized version of alienation and digital exploitation reinforces exploitative systems based on race, class, gender, and sexuality; a critical and intersectional framework is thus necessary to begin to challenge this system of exploitation.
Works Cited
Marx, K. (1844). Economic and philosophic manuscripts of 1844.
Öhman, C. Introducing the pervert’s dilemma: a contribution to the critique of Deepfake Pornography. Ethics Inf Technol 22, 133–140 (2020). https://doi.org/10.1007/s10676- 019-09522-1
Wagner, T. & Blewer, A. (2019). “The Word Real Is No Longer Real”: Deepfakes, Gender, and the Challenges of AI-Altered Video. Open Information Science, 3(1), 32-46. https://doi.org/10.1515/opis-2019-0003