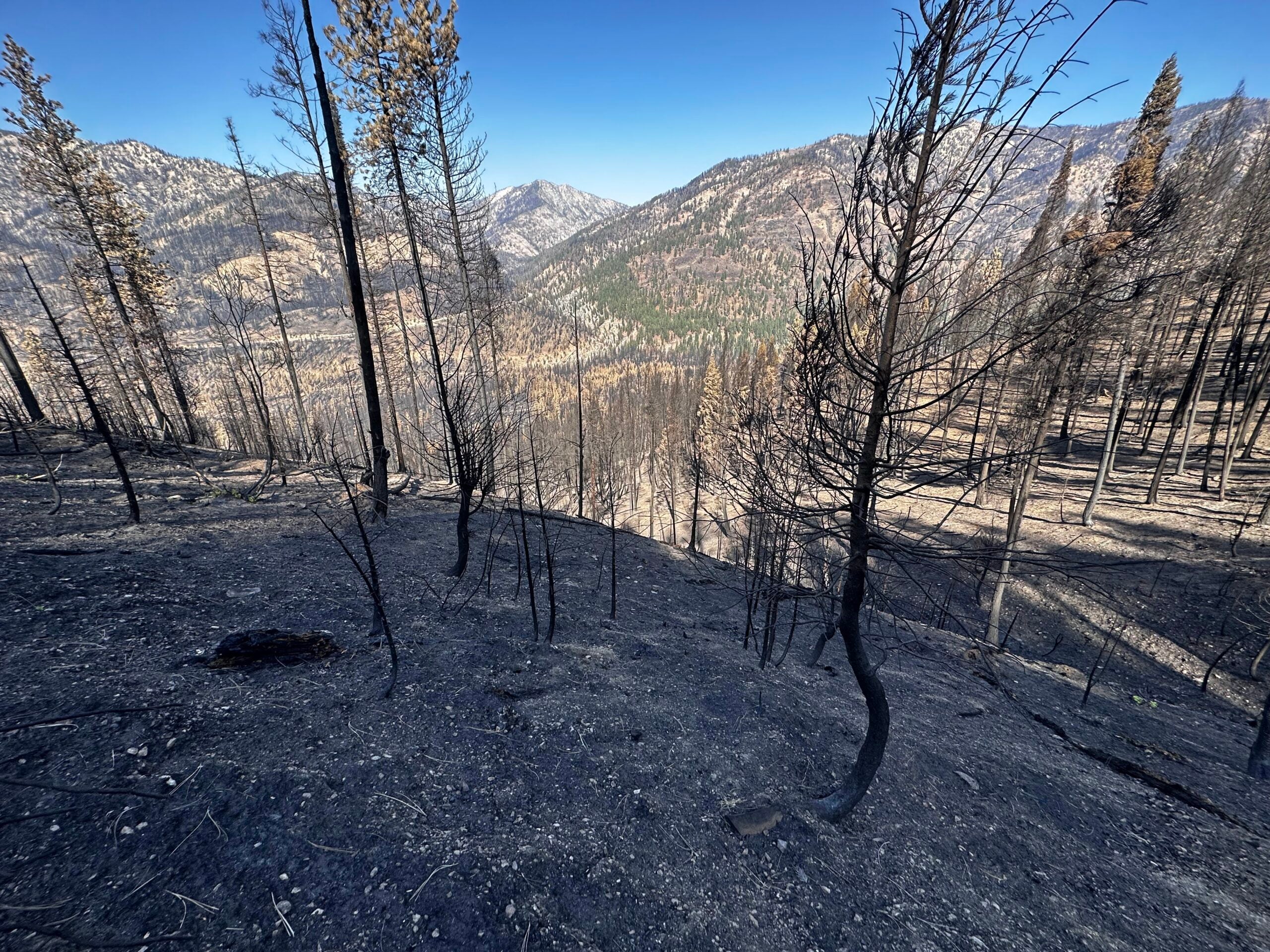
An understanding of second and third-degree burns on human tissue may be common, but what happens when wildfire scorches the soil? Though the earth isn’t flesh and bone, it too can burn at varying degrees of severity, leading to changes or loss of organic matter above and below ground.
Wildfires not only leave behind thick layers of ash and combusted material, but also destroy the plants whose roots anchor the soil and prevent erosion. Add a rain shower to this mix, and it creates the perfect recipe for costly and dangerous debris flows, or mudslides. A heart wrenching example is the 2018 Montecito debris flow in California that claimed 23 lives.
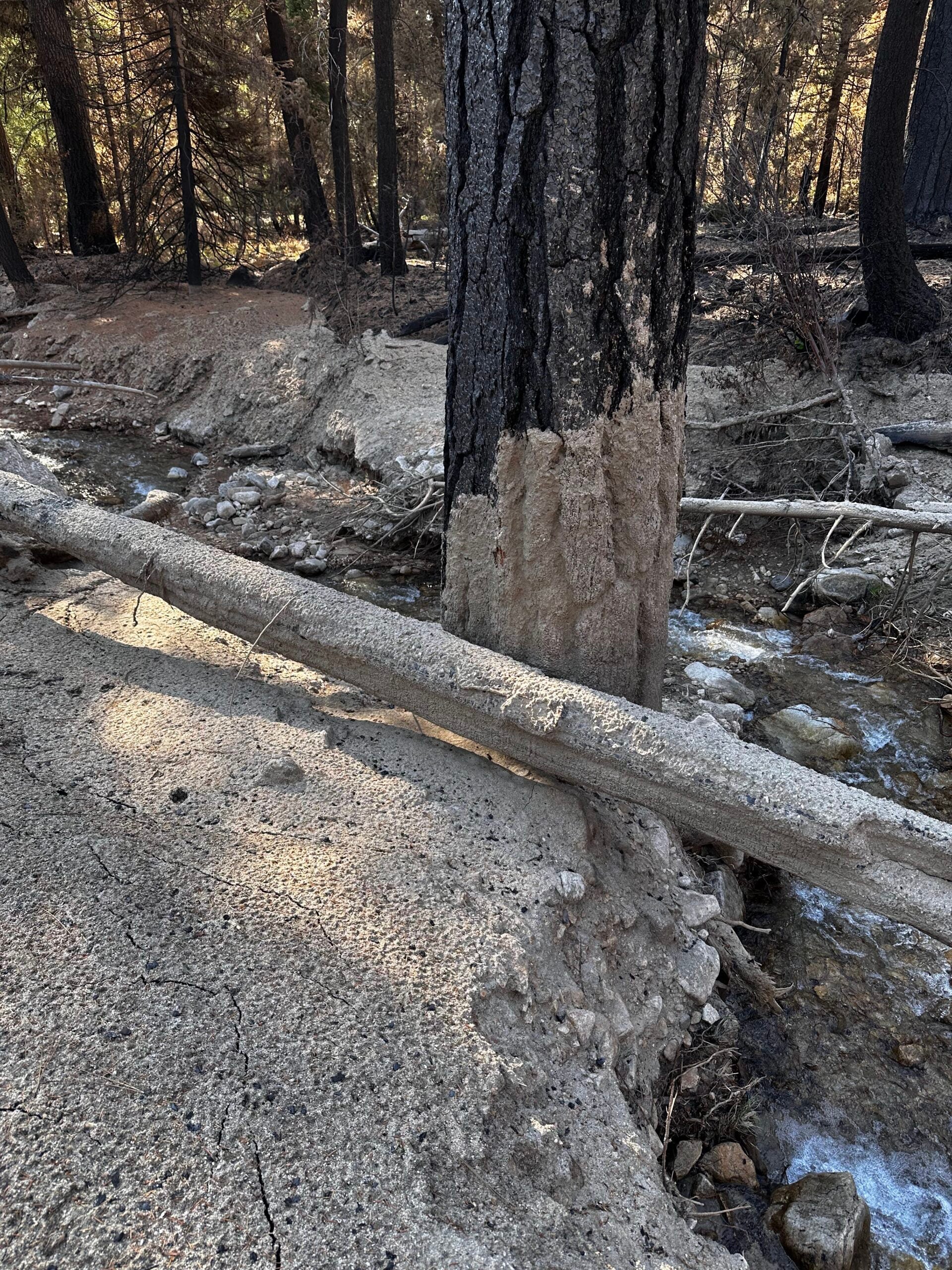
Discovering the level of burn severity in post-wildfire areas is crucial. Currently, researchers use satellite data of vegetation to measure burn severity, but this data can only show what’s happened to the above-ground vegetation. This approach must be followed up with trekking miles of difficult and often dangerous terrain to collect ash, soil and water samples and recalibrate their field maps. Even with this hard work, these burn severity maps can only paint a fragment of the total picture.
School of Computing doctoral student Amirhossein (Amir) Montazeri has a plan to change that using his expertise in data science, hydrology engineering and a prestigious Future Investigators in NASA Earth and Space Science and Technology award.
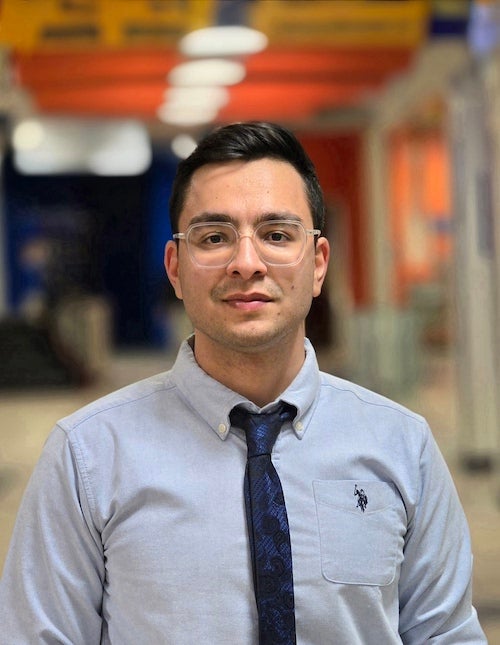
“Fires can markedly alter the watershed hydrology, geology, and the surface of the earth, so it can easily cause floods, debris flows, water quality problems, erosion and damage and contaminate the downstream infrastructures like reservoirs. So it’s important to measure and address those effects,” Montazeri said.
Under the mentorship of Mojtaba Sadegh, an associate professor of civil engineering, Montazeri will use data from many sources – such as weather, vegetation indices, soil characteristics and large fire incidents across the western U.S. – and artificial intelligence techniques to develop a predictive understanding of how ecological, meteorological, climatic, geological and topographical processes prior to and during wildfires shape soil burn severity data outcomes.
The goal is to create a physics-informed machine learning model of soil burn severity that not only predicts wildfire impacts on soil and informs post-fire hazard mitigation, but also answers fundamental scientific questions about the amount of control various processes have on soil burn severity outcomes.
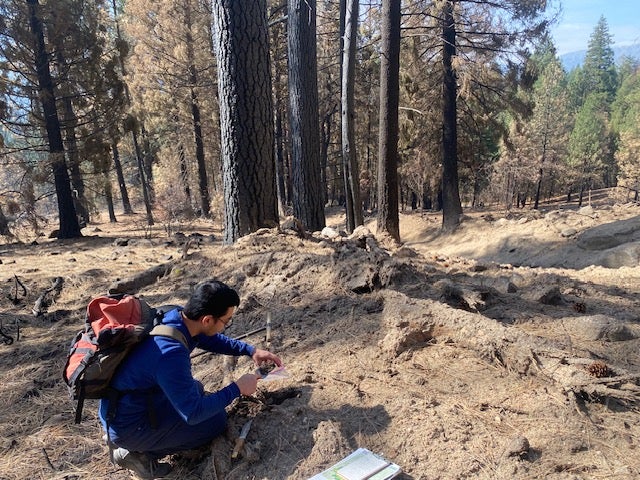
While developing this algorithm, Montazeri had the opportunity to join geosciences professor Jen Pierce and a team of researchers collecting soil burn severity samples after the 2024 Wapiti Fire burned in Idaho.
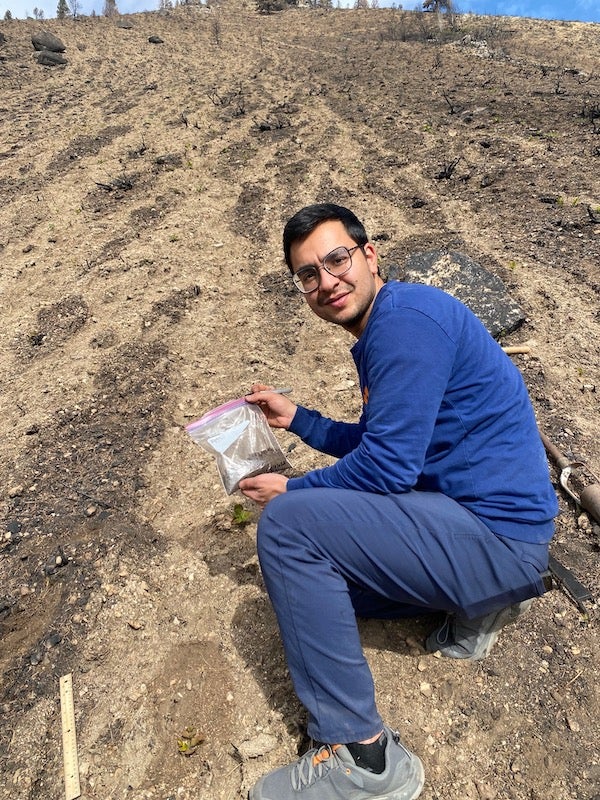
For Montazeri, experiencing the sample collection process across miles of burned Idaho forest and speaking to home-owners and business leaders who were impacted was profound. This has only fueled his desire to make the process of creating soil burn severity maps safer and more efficient.
“I just wanted to know everything about it and observe what’s going on in a real situation to make better predictions with my models,” Montazeri said. “I knew that the fire was severe and it would affect the downstream systems, but I can’t believe how big it was. You talk with some of the people that are living downstream after the rain caused destruction of the roads, the electricity…it became tangible for me when I saw it.”