Imagine it is the dead of night and a wildfire starts without anyone present to witness its origin. Was it an ember from a campfire? A stray firework? A spark from a power line? A car backfiring? Arson?
Currently there are 12 known forms of human-started ignition. The 13th is simply categorized as ‘unknown’. Unfortunately, the origins of more than 50% of reported fires in recent years fall under this category, and that’s a serious problem. Understanding the origins of a fire is essential for authorities and fire management teams to determine their response, especially as climate change makes wildfires a dangerous and expensive certainty.
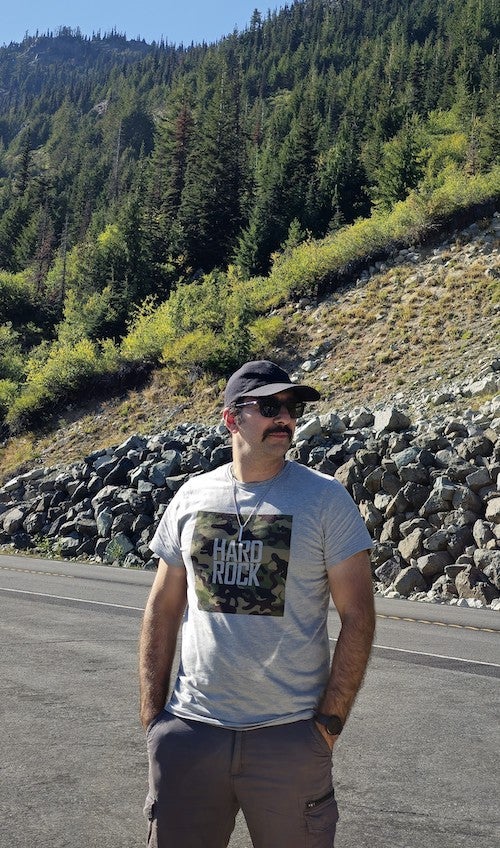
But what if someone could revolutionize wildfire categorization by harnessing machine learning and be able to provide–for the first time–the probable cause of every unknown fire ignition?
Enter Yavar Pourmohamad. Pourmohamad is a doctoral student in the School of Computing working alongside Civil Engineering faculty member Mojtaba Sadegh, and in January 2025, he published groundbreaking research highlighting the promise of machine learning in the battle against wildfire origin categorization.
In the study, Pourmohamad developed a machine learning algorithm taking into account 39 unique features–such as weather data, topography, land usage, social factors, and more– to analyze the 150,247 wildfires ‘unknown’ wildfires that burned in the Western U.S. from 1992 to 2020.
Pourmohamad discovered that the model more accurately separated wildfires ignited by natural versus human causes (with 93% accuracy), and discriminated among the 11 classes of human-ignited wildfires with 55% accuracy. In the model, the team also discovered that the greatest percentage ‘unknown’ ignition source could be correlated to equipment and vehicle use (21%), lightning (20%), and arson and incendiarism (18%).
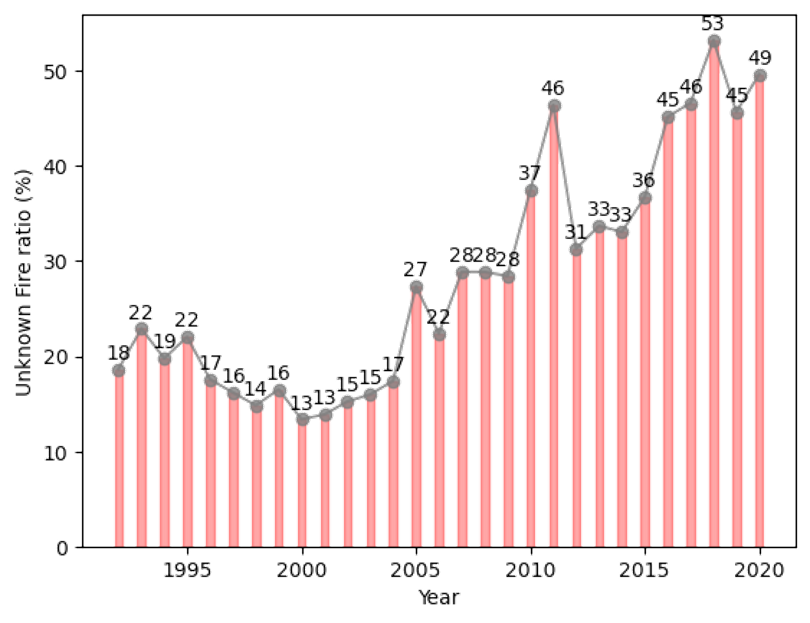
With students in the computer science program, Pourmohamad also created a Boise State-hosted web application that visualizes individual fire incidents from 1992-2020 and their attributes – including location, date, size and cause, as well as social, biophysical, and biological characteristics—from the Fire Program Analysis – Fire Occurrence Database Attributes dataset. The next step for the website is adding in the machine learning component so users can see the probability of wildfire ignition on their selected day and location.
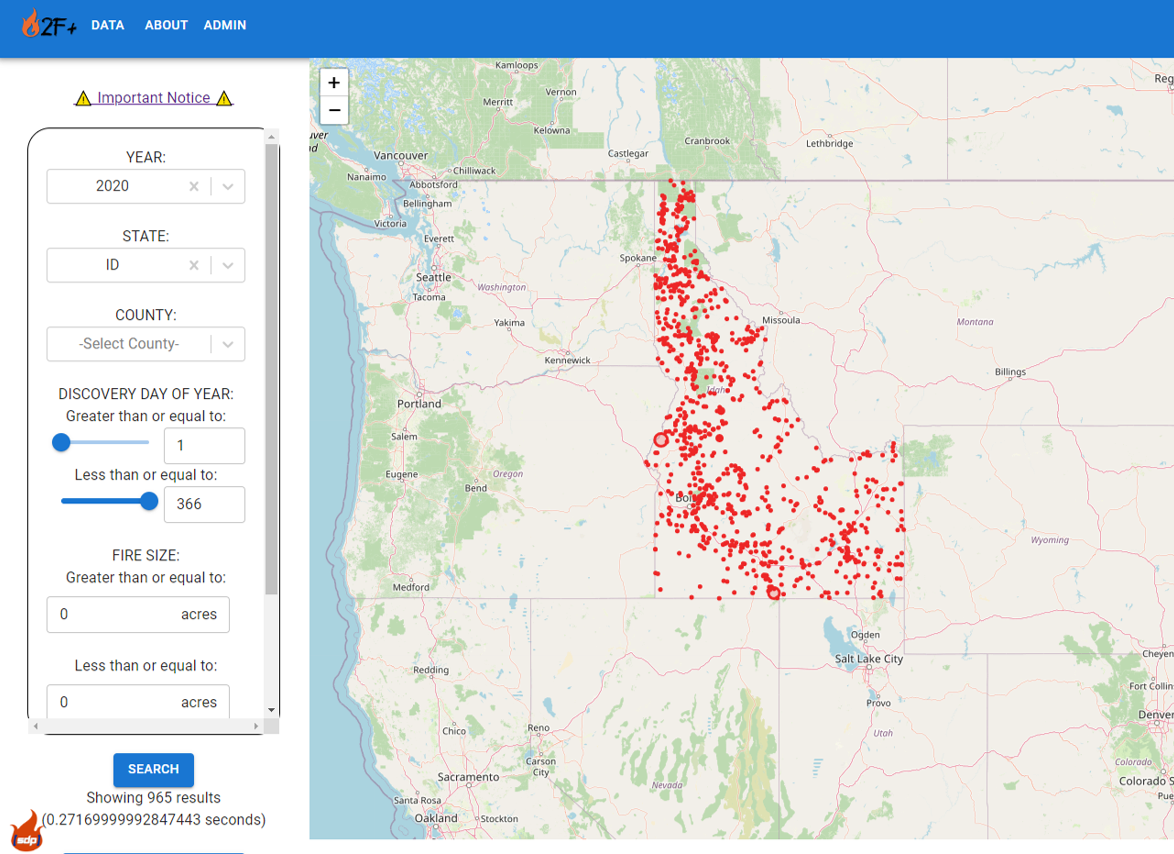
Knowledge resulting from this study can help authorities shapes policies in a way that could prevent fires, save the lives of civilians and emergency personnel, as well as saving untold dollars in property damage.
“This is the part that the university and researchers can actually contribute to help them to come up with a better solution,” Pourmohamad said.
“This is one of my life goals, and even if I leave Boise State, every time that I see this website gets something added to it, I would be thrilled. This is something that I’m going to show my children, and say ‘Hey, see, this is what I did when I was at your age!”