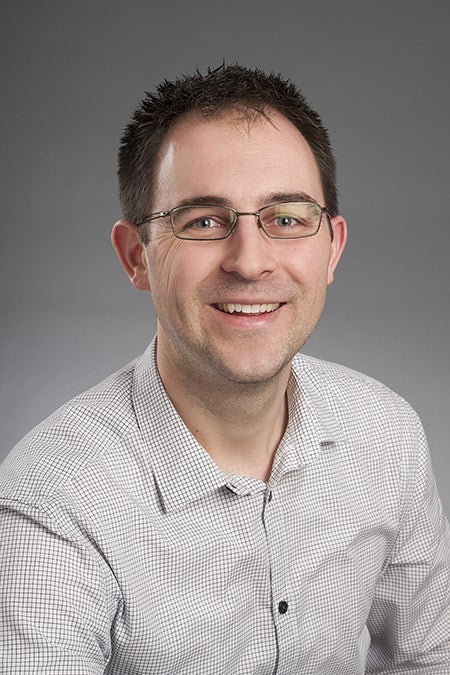
Kurtis Cantley, associate professor of electrical and computer engineering, will conduct machine learning hardware research with a three-year grant of $700,000 from the Department of Energy Established Program to Stimulate Competitive Research (EPSCoR). The goal of Cantley’s research will be to improve the reliability of the power grid with intelligent, adaptable and cyber secure electronics that take inspiration from the human brain, otherwise known as neuromorphic systems.
“Machine learning and artificial intelligence algorithms are typically implemented in software running on digital computers,” Cantley said. “When we talk about neuromorphic systems, that means it’s basically machine learning done in hardware, or an electronic system that is specifically meant to implement machine learning algorithms really efficiently.”
This award is part of a larger $21 million initiative by the Department of Energy to support energy-relevant research in underrepresented regions. With the grant, Cantley will collaborate with Idaho National Laboratory to develop novel neuromorphic hardware to monitor power grid systems. This research will not only improve the computational efficiency of power-grid monitoring systems, but the novelty of this hardware will make it considerably more complicated for cyber attackers to reverse engineer than standard off-the-shelf hardware.
Power systems are already incredibly complex, Cantley says, and they are increasingly becoming even more complicated. Everything from home solar grids, to enormous factories, to smart device microgrids all need reliable energy delivery. Reliability requires intelligent monitoring that can identify even the slightest anomalies and respond accordingly. That’s where neuromorphic systems could make a difference.
“We will mostly conduct hardware simulation, but there is some money in this grant to actually design and have our own chips fabricated to implement the algorithms that we develop for monitoring subsystems of the power grid,” Cantley said.
With these simulations and hardware, Cantley and his team will be able to test the neuromorphic system’s ability to monitor the power grid subsystem for a small neighborhood-sized area. However, the neuromorphic approach could easily scale to much larger areas in the future.