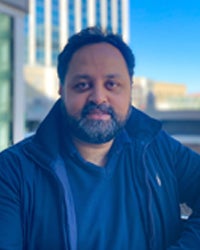
Amir Abbas Kazemzadeh had some experience with machine learning before enrolling at Boise State, but it was not until he co-authored a grant proposal with his now advisor, Dr. Mahmood Mamivand, that it became a passion. This partnership is what brought Amir to the Computing Ph.D. program and sparked his interest in using machine learning to change material design.
The Minerals, Metals, and Materials Society (TMS) hosts an annual meeting each year to showcase advancements made in the field. Amir submitted abstracts for a couple of the symposiums that were happening at the event at the recommendation of his advisor. After submitting his work, he was invited to present at both symposiums in Anaheim, California. Amir commented that his favorite part of the experience was meeting with pioneers in the field. “I have already studied their papers, and it was great to meet them. I could talk with them about my projects and get their comments.” This also helped Amir network in preparation for post-graduation research positions.
Amir continues to focus his research on using machine learning and data science in material science as he completes his Ph.D. He hopes to get a job as a data scientist in the automation or process optimization industries after graduation. In his free time, Amir likes to hike, listen to podcasts, and read historical or biographical books.
Below you will find the abstracts Amir presented at the TMS annual meeting:
Chemistry and Processing History Prediction from Materials Microstructure by Deep Learning
Abstract
Finding the chemical composition and processing history from a microstructure morphology for heterogeneous materials is desired in many applications. In this work, we develop a framework based on a deep learning approach that enables us to predict the chemistry and processing history just by reading the morphological distribution of one element. We used a dataset from spinodal decomposition simulation of FeCrCo alloy created by the phase-field method as a case study. We develop specific algorithms to manage the mixed dataset, including both images and continuous data. Results show that while shallow networks are efficient in chemistry prediction, deep networks are required to predict the processing temperature accurately. The physical concepts behind these observations will be discussed. The results also show that transfer learning outperforms the in-house trained network when it comes to microstructure features extraction.
Spatiotemporal Prediction of Microstructure by Deep Learning
Abstract
Prediction of microstructure evolution during material processing is essential to control the material properties. Simulation tools for microstructure evolution prediction based on physical concepts are computationally expensive and time-consuming. Therefore, they are not practical when there is an urgent need for microstructure during the process. Essentially, microstructure evolution prediction is a spatiotemporal sequence prediction problem, where the prediction of material microstructure is difficult due to different process history and chemistry. We propose a recurrent neural network for spatiotemporal predictive learning (PredRNN) model for the microstructure evolution prediction. As a case study, we used a dataset from spinodal decomposition simulation of FeCrCo alloy created by the phase-field method for training and predicting the future microstructures by previous observations. The results show that the trained network is capable of efficient prediction of microstructure evolution.